When Can Statistics Not Be Applied in Business? Exploring Limitations and Pitfalls
In the fast-paced world of business, data reigns supreme. Companies rely on statistics to make informed decisions, forecast trends, and optimize operations. However, there are critical moments when the application of statistics can lead to misleading conclusions or ineffective strategies. Understanding these limitations is essential for any business leader who aims to harness the power of data without falling into common traps. In this article, we will explore the scenarios in which statistics may not be applicable or effective, shedding light on the nuances that can impact decision-making.
Statistics is often viewed as a universal tool for analysis, but its efficacy can diminish in certain contexts. For instance, when data is sparse or not representative of the larger population, statistical methods may yield unreliable results. Additionally, the misuse of statistical techniques—such as cherry-picking data or misinterpreting correlations—can lead to erroneous conclusions that misguide business strategies. This highlights the importance of not only understanding statistical methods but also recognizing their limitations in real-world applications.
Moreover, the dynamic nature of business environments introduces further complexities. Rapid changes in market conditions, consumer behavior, or technological advancements can render statistical models obsolete or irrelevant. In such cases, relying solely on historical data may obscure emerging trends and hinder a company’s adaptability. By examining these scenarios, we can better appreciate
Inadequate Data Quality
Statistics rely heavily on the quality of data collected. If the data is flawed—whether due to errors in collection, processing, or recording—it can lead to misleading conclusions. In such cases, applying statistical methods can yield results that do not accurately reflect reality.
Factors contributing to inadequate data quality include:
- Sampling Bias: When the sample does not accurately represent the population, statistical results can be skewed.
- Measurement Errors: Inaccurate data collection methods can introduce errors that affect outcomes.
- Incomplete Data: Missing data points can lead to incorrect analyses and unreliable conclusions.
To illustrate the impact of data quality, consider the following table:
Data Quality Issue | Potential Impact on Analysis |
---|---|
Sampling Bias | Leads to non-representative samples, distorting results. |
Measurement Errors | Causes inaccuracies in data that can mislead interpretations. |
Incomplete Data | Results in gaps that can skew statistical outcomes. |
Non-randomized Experiments
In business contexts, non-randomized experiments can present significant challenges in applying statistical methods effectively. When participants in a study or experiment are not randomly assigned, it becomes difficult to establish causation, as other variables may confound results.
Key issues in non-randomized experiments include:
- Selection Bias: Participants may possess characteristics that influence the outcome, leading to skewed results.
- Confounding Variables: External factors can affect the results, making it hard to isolate the effect of the treatment or intervention.
To address these issues, businesses may need to consider alternative methods, such as observational studies or advanced statistical techniques that can help control for confounding variables.
Small Sample Sizes
Statistical methods often assume that sample sizes are adequate to draw valid conclusions. Small sample sizes can lead to unreliable results, as they may not adequately capture the variability of the population.
Challenges associated with small sample sizes include:
- Increased Variability: Results from small samples are more susceptible to fluctuations, leading to misleading interpretations.
- Reduced Statistical Power: The ability to detect a true effect diminishes with smaller samples, increasing the likelihood of Type II errors.
For effective analysis, businesses should aim for larger sample sizes whenever possible to enhance reliability and validity.
Dynamic and Rapidly Changing Environments
In highly dynamic business environments, statistics can become less applicable. Rapid changes can render historical data obsolete, making it challenging to rely on past trends to forecast future performance.
Considerations in dynamic environments include:
- Market Volatility: Sudden changes in consumer preferences can invalidate previous data analyses.
- Technological Advancements: New technologies can disrupt existing markets, making historical data less relevant.
In such cases, businesses may need to adopt more agile analytical approaches that incorporate real-time data and continuous monitoring to make informed decisions.
Limitations of Statistical Application in Business
Statistical methods are powerful tools for decision-making in business; however, there are specific scenarios where their application may be inappropriate or misleading. Understanding these limitations is crucial for effective data analysis and interpretation.
Insufficient Data
Statistics rely heavily on the quality and quantity of data. When data is scarce or insufficient, the results can be unreliable. Key issues include:
- Small Sample Size: Results drawn from a small sample may not accurately represent the broader population.
- Biased Data Collection: If the data collection method is biased, it can skew results and lead to incorrect conclusions.
For example, a market survey based on responses from only a specific demographic may not reflect the preferences of the entire customer base.
Non-Normal Distributions
Many statistical techniques assume that the data follows a normal distribution. If this assumption is violated, the results can be misleading. Consider the following:
- Skewed Data: When data is heavily skewed, measures such as mean can be misleading.
- Outliers: Extreme values can disproportionately influence statistical calculations, leading to erroneous interpretations.
In such cases, non-parametric methods or transformations may be necessary.
Overfitting Models
In statistical modeling, overfitting occurs when a model captures noise instead of the underlying trend. This can lead to:
- Poor Predictive Power: Models that are too complex may perform well on training data but fail to generalize to new data.
- Misleading Insights: Overfitted models can suggest relationships that do not actually exist.
To combat overfitting, practitioners can use techniques such as cross-validation and regularization.
Assumption Violations
Statistical methods often come with underlying assumptions. When these assumptions are violated, the validity of the results can be compromised. Common assumptions include:
- Independence of Observations: Data points should not influence one another. Violations can occur in time series data or clustered data.
- Homogeneity of Variance: Variability among groups should be similar. Heteroscedasticity can lead to inaccurate estimates of significance.
Identifying and addressing these violations is crucial for accurate analysis.
Qualitative Data Limitations
Statistical techniques are primarily designed for quantitative data. When dealing with qualitative data, the application of statistics can be limited:
- Categorical Data: Analysis is restricted to frequency counts and simple comparisons.
- Subjective Interpretations: Qualitative insights can be influenced by personal bias, making statistical validation challenging.
In such cases, qualitative analysis methods may be more appropriate.
Dynamic Market Conditions
Business environments are dynamic and can change rapidly. Statistical models based on historical data may become obsolete:
- Changing Consumer Preferences: Trends can shift, making past data irrelevant for current decision-making.
- Market Disruptions: Unexpected events (e.g., economic crises, technological advances) can render existing models ineffective.
To maintain relevance, businesses must continuously update their data and models.
Ethical Considerations
The application of statistics in business raises ethical concerns, particularly regarding data privacy and representation:
- Data Misuse: Inaccurate or manipulative use of statistics can lead to unethical business practices.
- Lack of Representation: Failure to include diverse populations in data collection can perpetuate biases.
Ethical considerations must be integrated into the statistical analysis process to ensure responsible decision-making.
Limitations of Statistical Application in Business
Dr. Emily Chen (Data Scientist, Business Analytics Institute). “Statistics cannot be effectively applied in business when the underlying data is biased or unrepresentative. If the sample does not accurately reflect the population, any statistical conclusions drawn will be misleading and can lead to poor decision-making.”
Mark Thompson (Senior Business Analyst, Market Insights Group). “In scenarios where the business environment is highly dynamic and unpredictable, such as during a major economic crisis, traditional statistical models may fail. These models often rely on historical data, which may not be applicable in rapidly changing conditions.”
Linda Garcia (Professor of Business Statistics, University of Commerce). “Statistics should not be applied in business when qualitative factors are paramount. For instance, in assessing employee satisfaction or customer loyalty, relying solely on quantitative metrics without understanding the qualitative context can lead to misguided strategies.”
Frequently Asked Questions (FAQs)
When can statistics not be applied in business decision-making?
Statistics may not be applicable in business decision-making when data is insufficient, unreliable, or biased. Situations involving unique, non-recurring events also limit the utility of statistical analysis.
What are the limitations of using statistics in forecasting?
Statistics can be limited in forecasting when historical data is not indicative of future trends, such as in rapidly changing markets or during unprecedented events. Additionally, over-reliance on statistical models without considering qualitative factors can lead to inaccurate predictions.
In what scenarios might statistical assumptions be violated?
Statistical assumptions may be violated in cases of non-normal data distributions, heterogeneous variances, or when data points are not independent. Such violations can compromise the validity of statistical tests and conclusions.
How does small sample size affect statistical analysis in business?
A small sample size can lead to unreliable results, increased variability, and a higher risk of Type I and Type II errors. It limits the generalizability of findings and can produce misleading insights about the population.
Why is it important to consider context when applying statistics in business?
Context is crucial because statistical results can be misinterpreted without understanding the underlying business environment, market conditions, and external factors. Ignoring context can lead to erroneous conclusions and poor decision-making.
What are the risks of misusing statistics in business?
Misusing statistics can result in flawed strategies, misguided investments, and loss of credibility. It may also lead to ethical issues, particularly if data is manipulated to support a predetermined narrative or outcome.
In the realm of business, statistics serve as a vital tool for decision-making, analysis, and forecasting. However, there are specific scenarios where the application of statistical methods may be inappropriate or ineffective. These situations often arise due to issues such as insufficient data, the presence of bias, or the misuse of statistical techniques. Recognizing these limitations is crucial for business professionals to avoid erroneous conclusions that could lead to poor strategic decisions.
One significant instance where statistics may not be applicable is when the data collected is inadequate or unrepresentative of the population being studied. This can lead to skewed results and misinterpretations. Additionally, when data is influenced by biases—whether from sampling methods or data collection processes—the validity of statistical conclusions can be compromised. It is essential for businesses to ensure that their data is robust and representative to derive meaningful insights.
Moreover, the misuse of statistical techniques can also hinder effective application. For example, applying complex statistical models without a proper understanding of their assumptions can result in misleading outcomes. Businesses must be cautious in their approach to statistics, ensuring that the methods employed align with the nature of the data and the specific questions being addressed.
while statistics are a powerful asset for businesses, there
Author Profile
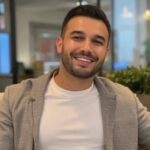
-
Alec Drayton is the Founder and CEO of Biracy, a business knowledge platform designed to help professionals navigate strategic, operational. And financial challenges across all stages of growth. With more than 15 years of experience in business development, market strategy, and organizational management, Alec brings a grounded, global perspective to the world of business information.
In 2025, Alec launched his personal writing journey as an extension of that belief. Through Biracy, he began sharing not just what he’d learned. But how he’d learned it through hands-on experience, success and failure, collaboration, and continuous learning. His aim was simple: to create a space where people could access reliable. Experience-driven insights on the many facets of business from strategy and growth to management, operations, investment thinking, and beyond.
Latest entries
- March 31, 2025Marketing & SalesDoes New Hampshire Impose Sales Tax on Car Purchases?
- March 31, 2025General Business QueriesHas the Professional Speaking Business Slowed Down: What Do the Trends Indicate?
- March 31, 2025General Business QueriesIs Dakota Arms Still In Business? Unraveling the Current Status of This Iconic Firearms Brand
- March 31, 2025Investment Queries And StrategiesIs Ocean Power Technologies a Smart Investment Choice in Today’s Market?